2025’s Data, Analytics & AI Trends
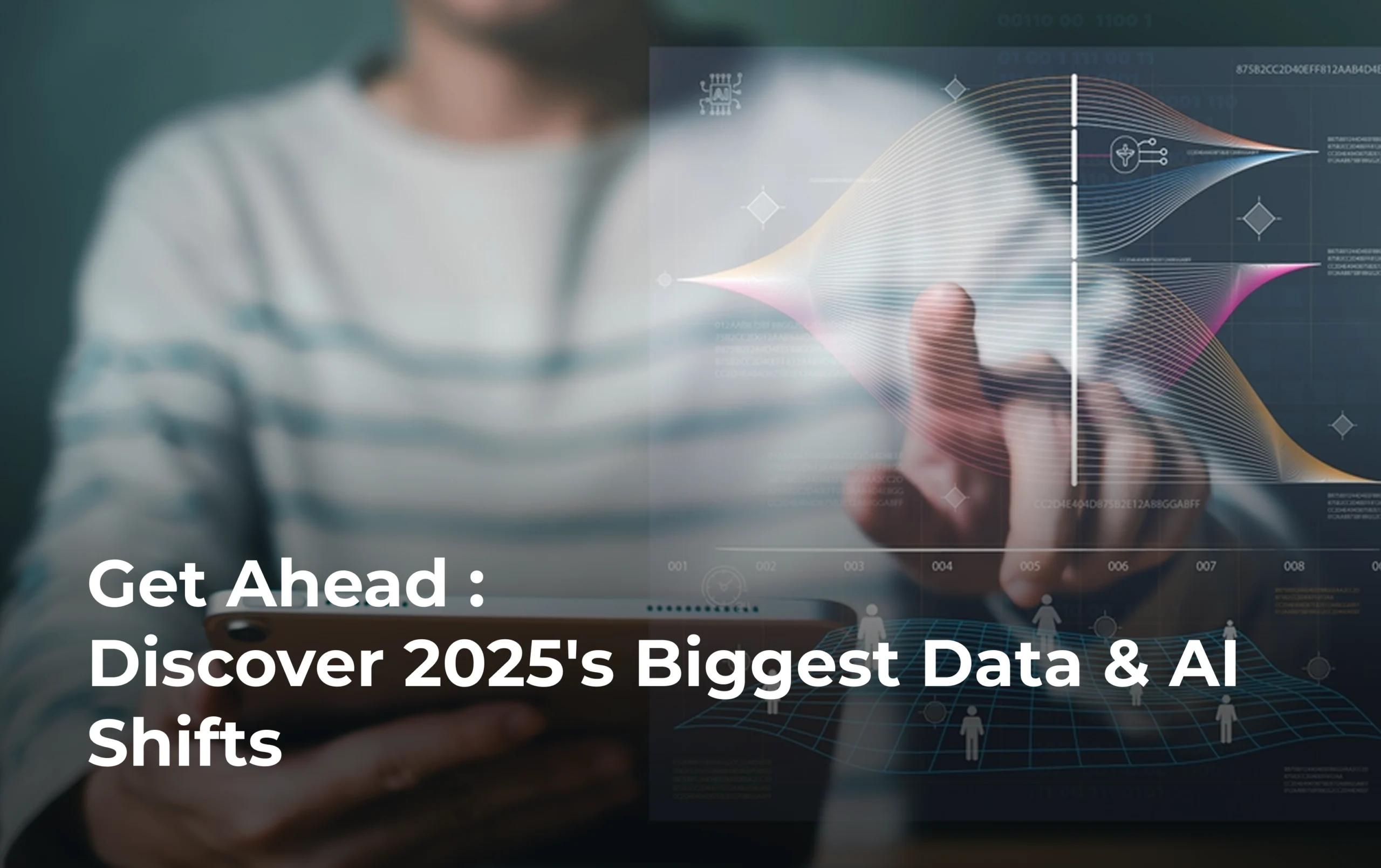
In a world where technological advancements unfold at a breathtaking pace, simply keeping up is no longer sufficient. True leadership and sustainable success demand foresight – the ability to anticipate, understand, and harness the forces shaping tomorrow. For organizations navigating the complex digital landscape, the currents of Data, Analytics, and Artificial Intelligence (AI) are arguably the most powerful drivers of change. Staying ahead of these trends isn’t just a competitive advantage; it’s fundamental to survival and growth.
Welcome to your essential guide for 2025. The coming year promises not just incremental improvements but significant paradigm shifts in how we collect, process, interpret, and leverage data. From the rapid evolution of generative AI to sophisticated new approaches in causal reasoning and the rise of autonomous systems, the landscape is transforming. Understanding these shifts is the first step towards strategically positioning your organization to thrive in the data-driven future.
This report delves into 12 critical trends poised to redefine industries, reshape business models, and unlock unprecedented levels of innovation and efficiency. Whether you’re a C-suite executive charting the strategic course, a data scientist building the next generation of models, or an IT leader architecting the infrastructure of tomorrow, these insights will equip you to make informed decisions and lead the charge. Let’s explore the forces that will shape 2025 and beyond.
The 12 Trends That Will Shape the Future:
1. xLMs, What’s Next? Beyond the Textual Horizon
Large Language Models (LLMs) like ChatGPT captured the world’s imagination, demonstrating the power of AI to understand and generate human-like text. But 2025 marks a significant evolution beyond purely text-based interactions. The trend is moving towards “xLMs” – encompassing Extended, Expansive, and Multimodal Large Models.
-
- Multimodal Integration: The next wave of LLMs will seamlessly integrate and reason across diverse data types – text, images, audio, video, code, and even sensor data. Imagine an AI that can analyze a product design sketch (image), understand user feedback from call transcripts (audio), cross-reference technical specifications (text), and suggest improvements (text/image). This multimodal capability unlocks richer context and more nuanced understanding, enabling applications in areas like enhanced diagnostics in healthcare (analyzing medical images alongside patient notes), more immersive customer experiences (generating video responses based on text queries), and sophisticated content creation.
- Specialized Advancements: While general-purpose LLMs continue to improve, we’ll see a surge in highly specialized models trained on domain-specific data. These models will offer superior accuracy and deeper insights within specific fields like finance (FinLLMs), law (LegalLLMs), or scientific research (SciLLMs). This specialization allows for more reliable and contextually relevant outputs, moving beyond general knowledge to expert-level assistance.
- Efficiency and Accessibility: Research is intensely focused on making these powerful models more efficient to train and run, reducing computational costs and environmental impact. Techniques like model distillation, quantization, and optimized architectures will make advanced AI more accessible to a broader range of organizations, not just tech giants.
Impact:
The evolution to xLMs signifies a move towards AI that perceives and interacts with the world more like humans do. For businesses, this means more powerful tools for analysis, automation, and creative problem-solving, impacting everything from product development and customer service to scientific discovery and strategic planning. The challenge lies in managing the complexity, cost, and ethical considerations of these increasingly powerful models.
2. Causal Inference: Moving Beyond Correlation to Understand “Why?”
For years, analytics has excelled at identifying correlations – spotting patterns and predicting what might happen next based on historical data. However, correlation doesn’t equal causation. Knowing that sales increase when marketing spend goes up is useful, but knowing why and by how much specific campaigns drive sales is transformative. This is the domain of Causal Inference.
Causal Inference provides a mathematical framework to move beyond mere prediction and delve into the underlying cause-and-effect relationships within data.
It seeks to answer critical business questions like:
-
- “What would happen to customer retention if we implemented this new loyalty program?” (Counterfactual analysis)
- “Why did sales drop in this region last quarter?” (Root cause analysis)
- “Which specific intervention – price discount or feature upgrade – will have a greater impact on user adoption?” (Treatment effect estimation)
By employing techniques like randomized controlled trials (A/B testing done right), propensity score matching, instrumental variables, and structural equation modeling, organizations can gain a deeper, more reliable understanding of the levers that truly drive outcomes.
Impact:
Causal Inference empowers organizations to make smarter, evidence-based decisions. Instead of relying on intuition or potentially misleading correlations, businesses can design more effective strategies, optimize resource allocation, and accurately measure the impact of their initiatives. This shift from predictive analytics (what will happen) to causal analytics (what will happen if we do X, and why) is crucial for driving measurable business value and achieving strategic goals. It requires a shift in mindset and potentially new skillsets within data science teams, but the payoff in decision quality is immense.
3. StAI Safe: Fortifying the Foundations of Generative AI
The explosive growth of Generative AI (GenAI) has brought incredible capabilities but also significant risks: misinformation, bias amplification, intellectual property concerns, security vulnerabilities, and a lack of transparency. The “StAI Safe” trend underscores the critical need for robust Governance, Security, and Ethical Frameworks to ensure responsible AI innovation.
As GenAI becomes more deeply embedded in business processes and customer interactions, establishing trust is paramount.
This involves:
-
- Robust Governance: Defining clear policies for AI development and deployment, including data usage rights, model validation procedures, acceptable use cases, and accountability structures. Security Frameworks: Protecting AI models from adversarial attacks (e.g., prompt injection, data poisoning), ensuring the confidentiality and integrity of training data, and securing the infrastructure running AI applications.
- Ethical Guardrails: Proactively addressing bias in training data and model outputs, ensuring fairness across different user groups, implementing explainability techniques (XAI) to understand model decisions, and aligning AI behavior with human values.
- Compliance: Navigating the evolving regulatory landscape (like the EU AI Act) and ensuring AI systems meet legal and industry standards.
Tools and techniques like AI risk assessments, bias detection algorithms, synthetic data generation for fairness testing, and secure AI development lifecycles (AI SecOps) are becoming essential components of the AI toolkit.
Impact:
“StAI Safe” is not about stifling innovation but channeling it responsibly. Organizations that prioritize AI safety, ethics, and security will build greater trust with customers, employees, and regulators. This trust is the foundation for widespread adoption and realizing the long-term benefits of GenAI. Conversely, neglecting these aspects can lead to reputational damage, legal liabilities, and erosion of stakeholder confidence. Embedding safety and ethics from the outset is crucial for sustainable AI success.
4. Insights Engineering: From Static Reports to Dynamic Decision Intelligence
Traditional business intelligence often involves static dashboards and periodic reports, which can quickly become outdated in today’s fast-paced environment. Insights Engineering represents a fundamental shift towards creating dynamic, real-time, and context-aware decision intelligence systems, increasingly powered by Generative AI.
It’s an engineering discipline focused on building scalable and reliable systems that deliver actionable insights directly into the workflows where decisions are made.
Key characteristics include:
-
- Real-time Data Integration: Connecting to diverse, streaming data sources to provide up-to-the-minute understanding.
- AI-Powered Analysis: Utilizing ML and GenAI to automate complex analyses, identify anomalies, predict future trends, and generate narrative explanations of findings.
- Natural Language Interaction: Enabling users to query data and request insights using plain language, making data analysis accessible beyond expert analysts (e.g., “Show me the sales trend for Product X in the Northeast region over the last quarter and explain any significant dips”).
- Contextual Delivery: Embedding insights directly within business applications (CRM, ERP, collaboration tools) where users need them, rather than requiring them to switch to separate BI platforms.
- Automation: Automating the generation and distribution of alerts, reports, and recommendations based on predefined triggers or detected patterns.
Impact:
Insights Engineering transforms data from a passive resource into an active partner in decision-making. It democratizes access to sophisticated analytics, accelerates the speed of insight generation, and empowers business users at all levels to make more informed, timely decisions. This leads to increased operational efficiency, improved agility, and a stronger data-driven culture throughout the organization.
5. Autonomous Data Constructs: Towards Self-Managing Data Ecosystems
Managing complex data ecosystems – pipelines, storage, quality checks, governance – traditionally requires significant manual effort from data engineers and operations teams. Autonomous Data Constructs leverage AI and automation to create data systems that can largely manage themselves.
These AI-driven systems aim to automate various aspects of the data lifecycle:
-
- Automated Data Pipelines: AI algorithms can design, build, monitor, and optimize data pipelines based on requirements, automatically adapting to changes in source data or target systems.
- Intelligent Data Quality: AI can automatically detect anomalies, profile data, suggest data quality rules, and even perform automated data cleansing and remediation tasks.
- Self-Healing Systems: Systems that can automatically detect failures (e.g., a broken pipeline, degraded performance) and initiate corrective actions without human intervention.
- AI-Driven Data Cataloging & Discovery: Automating the process of discovering, classifying, tagging, and documenting data assets, making it easier for users to find and understand the data they need.
- Optimized Resource Allocation: AI can dynamically manage storage tiers, compute resources, and data placement to optimize performance and cost.
Impact:
Autonomous Data Constructs promise to dramatically reduce the operational burden of managing data infrastructure. This frees up valuable data engineering talent to focus on higher-value activities like building new data products and enabling advanced analytics. It leads to faster data delivery, improved data reliability and quality, enhanced scalability, and potentially significant cost savings. While full autonomy is still evolving, the trend towards increasingly automated and intelligent data management is undeniable.
6. Bring Your Own Cloud (Interpreted via Prompt’s MAS Description): Multi-Agent Systems for Distributed Problem Solving
Initial Note: The prompt links “Bring Your Own Cloud” directly to a description of Multi-Agent Systems (MAS). While “Bring Your Own Cloud” (BYOC) typically refers to data sovereignty and hybrid/multi-cloud strategies allowing organizations to use their preferred cloud vendor within another platform, we will address the trend as described in the prompt, focusing on MAS.
Multi-Agent Systems (MAS) represent a cutting-edge AI approach moving beyond monolithic AI models. Instead of a single, large AI tackling a problem, MAS involves a collection of autonomous, interacting “agents.” Each agent might have specialized skills, goals, and knowledge, and they collaborate, negotiate, and coordinate to solve complex problems that might be intractable for a single entity.
Key characteristics of MAS include:
-
- Decentralization: Control and intelligence are distributed among agents, enhancing robustness and scalability.
- Collaboration & Negotiation: Agents interact, share information, and potentially negotiate to achieve individual or collective goals.
- Specialization: Individual agents can be optimized for specific tasks or possess unique data access.
- Emergent Behavior: Complex, intelligent behaviors can arise from the interactions of relatively simple agents, leading to novel solutions.
- Adaptability: MAS can often adapt more dynamically to changing environments as individual agents adjust their behavior.
In the context implied by the prompt (linking MAS to BYOC), one could interpret this as the ability to deploy and manage these sophisticated, potentially distributed MAS within an organization’s own controlled cloud environment (their “Own Cloud”). This ensures data privacy, security, and customization while leveraging the power of collaborative AI.
Impact:
MAS offers a powerful paradigm for tackling complex, dynamic problems found in areas like supply chain optimization, traffic management, financial market modeling, distributed resource allocation, and even scientific discovery. By enabling collaboration and specialized roles among AI agents, MAS can lead to more efficient, resilient, and adaptable solutions. The potential ability to run these within a secure, private cloud environment (BYOC concept) addresses key enterprise concerns around control and security.
7. Business AIgents: The Rise of Hyper-Customized AI Assistants
While general-purpose AI assistants are becoming common, the next frontier is Business AIgents – hyper-customized AI agents designed for specific business tasks, roles, and workflows. These are not generic chatbots but tailored AI collaborators integrated deeply into organizational processes.
Think of specialized agents designed to:
-
- Automate Customer Service: Handling complex queries, personalizing interactions based on customer history, and escalating issues intelligently.
- Support Sales Teams: Researching leads, drafting personalized outreach emails, scheduling meetings, and providing real-time insights during calls.
- Assist R&D: Sifting through research papers, analyzing experimental data, suggesting hypotheses, and even aiding in code generation or debugging.
- Streamline Operations: Monitoring supply chains, optimizing logistics, predicting maintenance needs, and managing inventory.
- Empower HR: Screening resumes, scheduling interviews, onboarding new hires, and answering employee policy questions.
The key is hyper-customization. These agents are trained on company-specific data, understand internal jargon and processes, adhere to organizational policies, and integrate seamlessly with existing software stacks (CRM, ERP, project management tools).
Impact:
Business AIgents promise a significant boost in productivity and efficiency by automating repetitive tasks and augmenting human capabilities. They can empower employees to focus on more strategic, creative, and complex work. This trend drives the need for platforms that allow organizations to easily build, deploy, manage, and govern these specialized AI agents securely and effectively.
8. From Hype to RoAI: Quantifying the True Value of Generative AI
Generative AI exploded onto the scene with significant hype, but organizations are now moving into a phase of critical evaluation: demonstrating tangible Return on AI (RoAI). Measuring the value of GenAI goes beyond simple cost savings or task automation; it requires innovative approaches to capture both quantitative and qualitative benefits.
Challenges in measuring RoAI include:
-
- Intangible Benefits: How do you quantify enhanced creativity, improved employee morale (due to less drudgery), faster innovation cycles, or better brand perception?
- Attribution: It can be difficult to isolate the specific impact of a GenAI implementation from other concurrent business initiatives.
- Long-Term Value: Some benefits, like fostering a more innovative culture, may only materialize over the long term.
Strategies for measuring RoAI include:
-
- Outcome-Based Metrics: Focusing on key business KPIs directly impacted by GenAI (e.g., sales conversion rates, customer satisfaction scores, time-to-market for new products, code commit frequency, bug reduction rates).
- Productivity Gains: Measuring time saved on specific tasks, reduction in manual effort, or increase in output for augmented roles.
- A/B Testing: Rigorously comparing processes or outcomes with and without GenAI intervention.
- Qualitative Assessments: Using surveys and interviews to capture perceived value, improvements in work quality, and employee/customer experience enhancements.
- Cost Avoidance: Calculating savings from prevented errors, reduced compliance risks, or optimized resource utilization.
Impact:
A clear focus on RoAI is essential for justifying continued investment in GenAI and scaling successful pilots across the organization. It moves the conversation from technological possibility to business value. Organizations need to establish clear goals, baseline metrics, and tracking mechanisms before deploying GenAI solutions to effectively demonstrate their impact and secure executive buy-in for future initiatives.
9. IBP Reloaded: Multi-Agent Systems Enhancing Business Planning
Note: This trend again leverages the concept of Multi-Agent Systems (MAS), applying it specifically to Integrated Business Planning (IBP).
Integrated Business Planning (IBP) aims to align strategic goals with operational capabilities across different business functions (sales, marketing, operations, finance). Traditionally, this complex process often struggles with data silos, conflicting objectives, and slow decision cycles. IBP Reloaded envisions leveraging Multi-Agent Systems (MAS) to revolutionize this process.
By applying the MAS paradigm (as described under Trend #6) to IBP:
-
- Simulating Complexity: Different agents can represent different business units (e.g., a Sales Agent, an Operations Agent, a Finance Agent). These agents can simulate their respective domains, considering local constraints and objectives.
- Cross-Functional Optimization: Agents can interact and negotiate to find globally optimal plans that balance competing demands (e.g., maximizing sales revenue vs. minimizing inventory costs vs. ensuring production feasibility).
- Enhanced Forecasting: Agents can incorporate diverse data sources and specialized forecasting techniques relevant to their domain, leading to more accurate and robust demand and supply predictions.
- Scenario Planning & Resilience: MAS allows for rapid simulation of various “what-if” scenarios (e.g., supply chain disruptions, sudden demand shifts). Agents can collaboratively explore responses and identify resilient strategies.
- Adaptive Planning: The system can continuously monitor real-world execution against the plan, allowing agents to quickly adapt and re-plan as conditions change.
Impact:
Applying MAS to IBP can transform it from a periodic, often cumbersome process into a dynamic, intelligent, and continuous cycle. This leads to improved alignment across the organization, better resource allocation, enhanced agility in responding to market changes, more resilient supply chains, and ultimately, better financial performance. It represents a significant leap forward in strategic and operational planning capabilities.
10. AI & Data Stack Sentinel: Navigating the Exploding Tech Landscape
The ecosystem of AI and data technologies is exploding. New tools, platforms, frameworks, and architectural patterns emerge constantly. Making the right technology choices is critical for building effective, scalable, and future-proof data and AI capabilities, but navigating this complex landscape is challenging. The AI & Data Stack Sentinel (or Observatory) trend highlights the need for continuous monitoring, evaluation, and strategic decision-making regarding the technology stack.
This involves:
-
- Technology Scouting: Actively tracking emerging technologies, open-source projects, vendor offerings, and research breakthroughs in areas like vector databases, MLOps platforms, GenAI frameworks, data observability tools, and cloud data services.
- Comparative Analysis: Evaluating different tools and platforms based on performance, cost, scalability, integration capabilities, community support, and alignment with organizational needs and skills.
- Architectural Foresight: Understanding how different components fit together and designing flexible, modular architectures that can accommodate future changes and prevent vendor lock-in.
- Risk Assessment: Identifying potential risks associated with adopting new technologies (e.g., immaturity, lack of support, security vulnerabilities).
- Internal Knowledge Sharing: Creating mechanisms to share findings and best practices across different teams within the organization.
Impact:
A proactive approach to monitoring the AI and data stack enables organizations to make informed technology investments, avoid costly dead ends, leverage cutting-edge capabilities faster, and build a more robust and adaptable data infrastructure. It requires dedicated effort – perhaps a specialized team or function – to act as the organization’s “eyes and ears” on the rapidly evolving tech frontier, ensuring strategic alignment between technology choices and business goals.
11. Enterprise Cognitive OS: The Unifying Intelligence Layer
Imagine an operating system, not for a computer, but for the entire enterprise’s collective knowledge and intelligence. This is the vision behind the Enterprise Cognitive OS or Cognitive Platform. It represents a unifying architecture designed to integrate diverse data streams and apply advanced AI to enable holistic understanding and sophisticated decision-making across the organization.
Key components and capabilities include:
-
- Unified Data Access: Seamlessly connecting to and integrating data from all sources – structured databases, unstructured documents, emails, sensor data, logs, external feeds, etc. – creating a comprehensive knowledge graph or semantic layer.
- Advanced AI Core: Incorporating various AI techniques – Machine Learning, Natural Language Processing, Generative AI, Knowledge Representation, Reasoning engines – to process, analyze, and synthesize information.
- Contextual Understanding: Moving beyond simple data retrieval to understand the relationships, context, and implications of information across different domains.
- Complex Reasoning: Enabling the system to perform multi-step reasoning, answer complex questions, generate hypotheses, and support strategic decision-making.
- Orchestration: Serving as a central hub to coordinate various AI models, business agents, and analytical workflows.
- API-Driven Access: Providing standardized interfaces for various applications and users (human and AI) to interact with the platform’s capabilities.
Impact:
The Enterprise Cognitive OS promises to break down information silos and create a truly integrated intelligence capability. It can power a wide range of applications, from hyper-personalized customer experiences and intelligent automation to strategic foresight and complex problem-solving. It represents the pinnacle of data-driven transformation, creating an organization that can learn, reason, and adapt at scale. Building such a platform is a significant undertaking but offers potentially transformative competitive advantages.
12. Cohorts of AIs: Scaling Intelligence Through Collaboration
Note: This is the third trend description explicitly mentioning Multi-Agent Systems (MAS).
Building on the concepts introduced in Trends #6 and #9, Cohorts of AIs further emphasizes the power of Multi-Agent Systems (MAS), focusing particularly on their ability to achieve scalability, adaptability, and tackle complexity through collaboration.
Where single AI models might struggle with the scale or multifaceted nature of certain problems, deploying “cohorts” or teams of interacting AI agents offers several advantages:
-
- Scalability: Problems can be decomposed and assigned to different agents, allowing for parallel processing and handling of larger datasets or more complex simulations.
- Adaptability: A system composed of multiple agents can potentially adapt more gracefully to changes. If one part of the environment changes, only the relevant agents need to adjust, rather than retraining a monolithic model.
- Resilience: The failure of a single agent may not cripple the entire system if other agents can compensate or take over its tasks.
- Handling Complexity: MAS is well-suited for problems with interacting components, emergent phenomena, and distributed information, such as simulating financial markets, managing autonomous vehicle fleets, optimizing large-scale logistics networks, or coordinating smart grids.
- Fostering Collaboration (AI-AI and Human-AI): These cohorts can include different types of agents (analytical, generative, predictive) working together, and can also be designed to collaborate effectively with human experts, augmenting team capabilities.
Impact:
The “Cohorts of AIs” trend signifies a shift towards more distributed, collaborative, and resilient AI architectures. It unlocks the potential to address problems previously considered too large or complex for AI. This approach will be crucial for advancements in scientific research, large-scale system optimization, and creating truly intelligent, adaptive autonomous systems. It pushes the boundaries of what AI can achieve by leveraging the power of collective intelligence.
Navigating the Future: Your Path Forward
The 12 trends outlined above paint a picture of a future powered by increasingly intelligent, interconnected, and autonomous systems. From the nuanced understanding offered by xLMs and Causal Inference to the efficiency gains from Autonomous Data Constructs and Business AIgents, and the collaborative power of Multi-Agent Systems – the potential for transformation is immense.
However, harnessing this potential requires more than just awareness. It demands strategic action:
-
- Assess Your Maturity: Where does your organization stand concerning its data infrastructure, analytics capabilities, and AI adoption? Identify gaps and opportunities relative to these trends.
- Prioritize & Strategize: Not all trends will be equally relevant or immediately actionable. Identify which trends offer the most significant potential value for your specific business context and develop a phased adoption strategy.
- Invest in Talent & Skills: The skills needed to leverage these trends (e.g., causal inference expertise, AI ethics, MLOps, prompt engineering, MAS development) are evolving. Invest in training your existing workforce and acquiring new talent.
- Foster a Data Culture: Technology alone is not enough. Cultivate an organizational culture that values data-driven decision-making, experimentation, and continuous learning.
- Embrace Responsible Innovation: Embed principles of security, ethics, fairness, and transparency (“StAI Safe”) into your AI initiatives from day one. Build trust as you innovate.
- Focus on RoAI: Move beyond the hype. Define clear metrics and relentlessly focus on demonstrating the tangible business value derived from your data, analytics, and AI investments.
The future outlined by these 2025 trends is not a distant horizon; it’s rapidly approaching. Organizations that proactively engage with these shifts, experiment thoughtfully, and integrate these capabilities strategically will not only navigate the future but actively shape it. This guide provides the map – the journey of data-driven innovation is yours to embark upon.